January 9, 2020
Deep learning, 3D technology to improve structure modeling for protein interactions, create better drugs
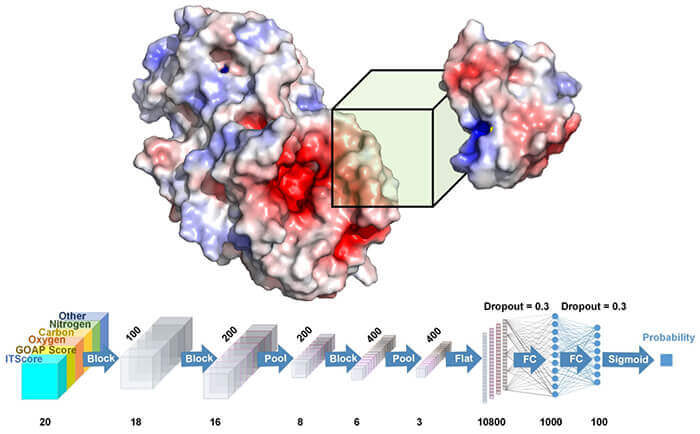
WEST LAFAYETTE, Ind. – Proteins are often called the working molecules of the human body. A typical body has more than 20,000 different types of proteins, each of which are involved in many functions essential to human life.
Now, Purdue University researchers have designed a novel approach to use deep learning to better understand how proteins interact in the body – paving the way to producing accurate structure models of protein interactions involved in various diseases and to design better drugs that specifically target protein interactions. The work is released online in Bioinformatics.
“To understand molecular mechanisms of functions of protein complexes, biologists have been using experimental methods such as X-rays and microscopes, but they are time- and resource-intensive efforts,” said Daisuke Kihara, a professor of biological sciences and computer science in Purdue’s College of Science, who leads the research team. “Bioinformatics researchers in our lab and other institutions have been developing computational methods for modeling protein complexes. One big challenge is that a computational method usually generates thousands of models, and choosing the correct one or ranking the models can be difficult.”
Kihara and his team developed a system called DOVE, DOcking decoy selection with Voxel-based deep neural nEtwork, which applies deep learning principles to virtual models of protein interactions. DOVE scans the protein-protein interface of a model and then uses deep learning model principles to distinguish and capture structural features of correct and incorrect models.
“Our work represents a major advancement in the field of bioinformatics,” said Xiao Wang, a graduate student and member of the research team. “This may be the first time researchers have successfully used deep learning and 3D features to quickly understand the effectiveness of certain protein models. Then, this information can be used in the creation of targeted drugs to block certain protein-protein interactions.”
Kihara has worked with the Purdue Research Foundation Office of Technology Commercialization on some of his research and technology.
About Purdue Research Foundation Office of Technology Commercialization
The Purdue Research Foundation Office of Technology Commercialization operates one of the most comprehensive technology transfer programs among leading research universities in the U.S. Services provided by this office support the economic development initiatives of Purdue University and benefit the university's academic activities through commercializing, licensing and protecting Purdue intellectual property. The office is managed by the Purdue Research Foundation, which received the 2019 Innovation and Economic Prosperity Universities Award for Place from the Association of Public and Land-grant Universities. For more information on licensing a Purdue innovation, contact the Office of Technology Commercialization at otcip@prf.org. The Purdue Research Foundation is a private, nonprofit foundation created to advance the mission of Purdue University.
Writer: Chris Adam, 765-588-3341, cladam@prf.org
Source: Daisuke Kihara, dkihara@purdue.edu
Abstract
Protein docking model evaluation by 3D deep convolutional neural networks
Xiao Wang, Genki Terashi, Charles W. Christoffer, Mengmeng Zhu, and Daisuke Kihara
Many important cellular processes involve physical interactions of proteins. Therefore, determining protein quaternary structures provide critical insights for understanding molecular mechanisms of functions of the complexes. To complement experimental methods, many computational methods have been developed to predict structures of protein complexes. One of the challenges in computational protein complex structure prediction is to identify near-native models from a large pool of generated models.
We developed a convolutional deep neural network-based approach named DOcking decoy selection with Voxel-based deep neural nEtwork (DOVE) for evaluating protein docking models. To evaluate a protein docking model, DOVE scans the protein–protein interface of the model with a 3D voxel and considers atomic interaction types and their energetic contributions as input features applied to the neural network. The deep learning models were trained and validated on docking models available in the ZDock and DockGround databases. Among the different combinations of features tested, almost all outperformed existing scoring functions.