Accelerating scientific and drug discovery in an AI-powered lab
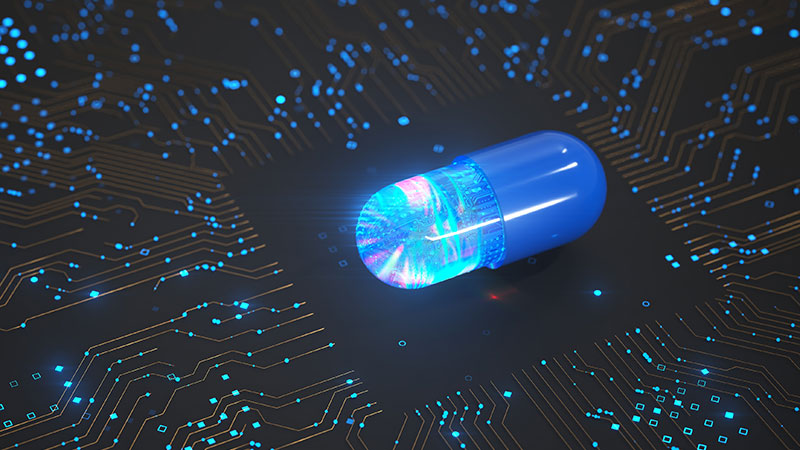
In Gaurav Chopra’s lab, discovery starts with a conversation with an artificial intelligence (AI) agent manager that helps to plan, execute and analyze experiments. Researchers chat in natural language and make a query, perhaps asking to design a drug for a particular target or synthesize a specific molecule. The tool — an AI agent manager — identifies all the resources to fulfill the task and communicates with lower-level agents that represent an instrument in the lab; or domain- or task-specific knowledge; or analysis AI and constructs a workflow to address the query given the resources available in the lab.
The AI agent manager plans the workflow to execute on instruments and identifies the procedures that will be part of the experiment. At the individual agent level, the workflow includes scheduling the instrument with appropriate settings and commands needed for execution and even running specific computer code for operating the instrument.
And then the work begins.
“The agent manager is like ChatGPT for the lab, and it works with the individual agents for each instrument or for domain-specific knowledge,” said Chopra, a Purdue associate professor of chemistry and (by courtesy) of computer science. “It’s a generalized, modular AI infrastructure that can do any task based on your objective. A lot of people are developing AI agents for one specific task. Our approach is task agnostic — I can swap out lab instruments or datasets and, if I do, I don’t have to change out the entire software infrastructure.”
Chopra is also a steering committee member for the Institute for Physical AI (IPAI) and core member of Regenstrief Center for Healthcare Engineering, and Purdue Institutes for Drug Discovery, Cancer Research and Integrative Neuroscience.
With the goal of accelerating scientific discovery, his lab has been developing the artificial intelligence/machine learning (AI/ML) infrastructure with the support of the National Institutes of Health National Center for Advancing Translational Sciences (NCATS). In 2022, Chopra and a team used the AI/ML infrastructure as part of a project that won the grand prize in the NCATS A Specialized Platform for Innovative Research Exploration (ASPIRE) competition. More recently, the NIH awarded a grant to Chopra’s lab to develop a blockchain-based, open science AI framework. The researchers will use blockchain-distributed ledger technology from AI technology developer Onai Inc. to protect IP-sensitive information to promote greater collaboration and reproducibility among different organizations and laboratories.
In a tongue-in-cheek reference to fictional AI developer Skynet, Chopra’s team calls the AI agent’s infrastructure SCINET (Scientific Communication Interaction NETwork). It runs on an “operating system” dubbed LINQX (Ledger for INteractive Query eXecution) that can be expanded or adapted to AI agent-based applications for any instrument or scientific discipline.
To incorporate a new instrument, for example, the team creates a new agent, which is a “living digital twin” of the instrument, with access to the instrument’s command libraries and the ability to learn from usage. The agent can physically control some instruments in the lab, but if the instrument commands are not integrated, the instrument can be manually operated with the AI agent offering instructions from an adjacent laptop. Chopra recently published a new automation workflow for lipidomics on arXiv with the support of instrument manufacturer Agilent Technologies Inc.
As it formulates its workflow, the AI agent manager presents its reasoning for the actions it proposes in a series of “thought, action, observation” steps generated based on the question and the resources available.
“The AI isn’t a black box, it shows you what it is thinking based on your question and what’s available to it,” Chopra said.
And Chopra said the developers in his lab — including graduate students Matthew Muhoberac, Connor Beveridge and Sanjay Iyer — want the AI to augment human effort.
“A lot of the efforts out there are trying to replace humans. We’re not trying to replace the human, we’re trying to augment their work. The whole system helps and works with the user, and plans things from a user perspective,” Chopra said. The human sets the path and the system accelerates workflows with datasets, computational models, accelerates analysis, accelerates experiments to be done based on the plan.”
At its current level, Chopra said SCINET also softens the learning curve for new students who may not know how to code, don’t know protocols for specific tasks, or have no experience in how to use or troubleshoot a particular instrument. Unlike other AI agents, the Chopra team has implemented a memory feature in which the system learns with each query and could eventually become a true research partner.
“The agents start off as technicians, but over time I think they will become scientists to help plan and execute the best way of doing these experiments on these instruments and develop new workflows to accelerate scientific and drug discovery with humans,” Chopra said.
Writer: Mary Martialay, mmartial@purdue.edu
Source: Gaurav Chopra, gchopra@purdue.edu