BIRCK BUILDS
THE FUTURE
The Birck Nanotechnology Center is home to Purdue’s advanced research and development on semiconductors and other technology at the atomic scale.
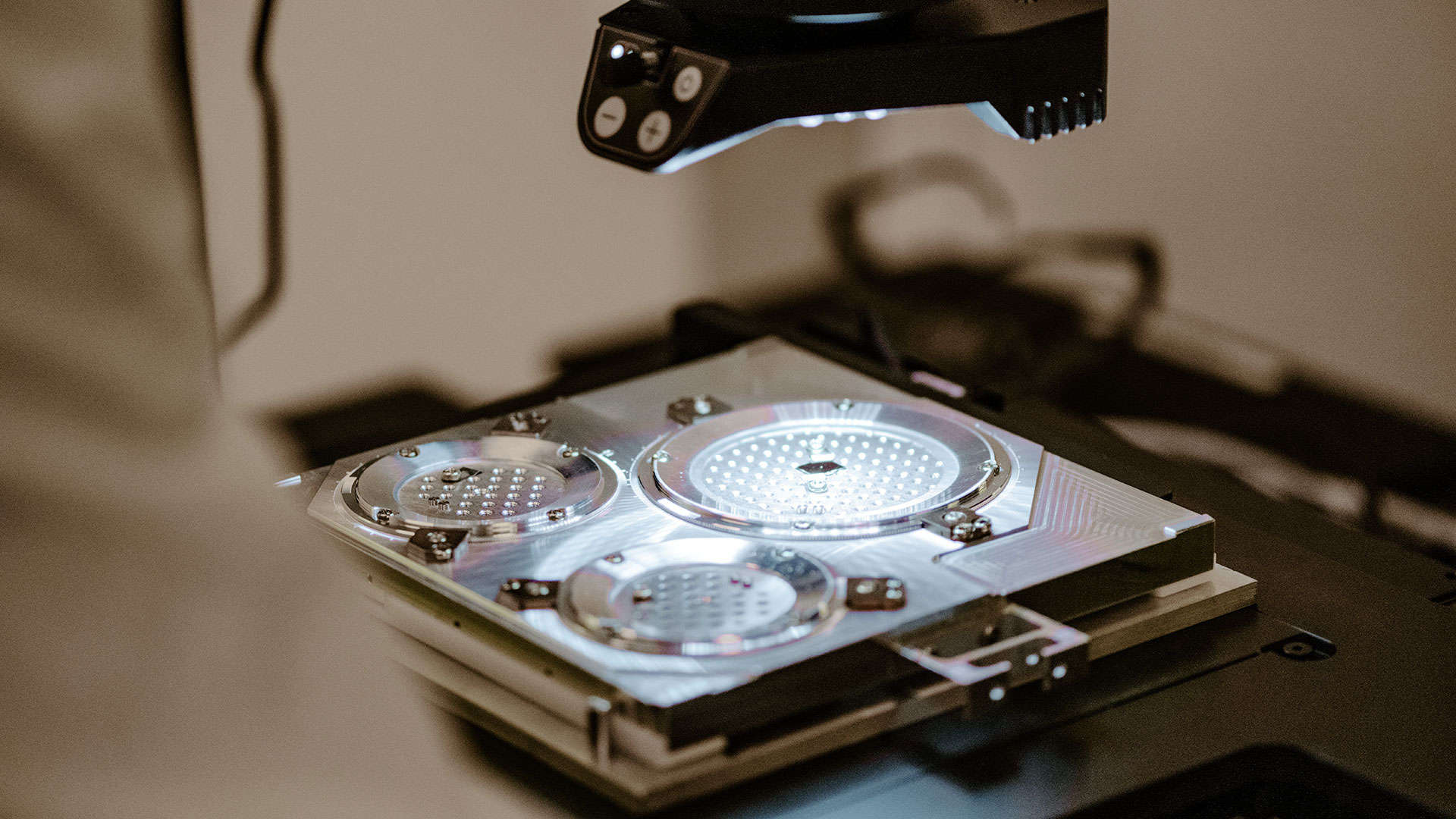
We Make It and Measure It
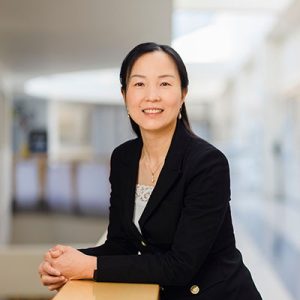
With the nation’s historic investments in nanotechnology and the strong commitment from Purdue’s leadership, I am confident that Birck will play an important role in educating the researchers and technical workforce of tomorrow and providing a platform for innovation and public/private partnership.
Zhihong Chen
The Mary Jo and Robert L. Kirk Director of the Birck Nanotechnology Center